Translate this page into:
The gamut of artificial intelligence in oculoplasty
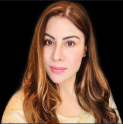
*Corresponding author: Kasturi Bhattacharjee, Department of Ophthalmology, Sri Sankaradeva Nethralaya, Guwahati, Assam, India. kasturibhattacharjee44@hotmail.com
-
Received: ,
Accepted: ,
How to cite this article: Bhattacharjee K, Rehman O, Sarkar A. The gamut of artificial intelligence in oculoplasty. J Ophthalmic Res Pract 2023;1:5-9.
Abstract
Artificial intelligence (AI) is taking its grasp over health-care system and ophthalmology as one of the most dynamic streams is largely influenced by AI. AI over the past few decades has made a huge impact in the bailiwick of oculoplasty. AI-based imaging softwares have made easier the diagnosis and management of several orbital and eyelid pathologies by its accuracy and reproducibility. AI also has made possible real-time tracking of deep orbital structures through navigation-guided technologies which have made orbital surgeries safer and easier. This article is a meta-analysis of several articles which have discussed applications and impact of AI-based software in diagnosis and management planning of periorbital and eyelid pathologies and also articles on navigation-guided orbital surgeries.
Keywords
Orbital imaging
Eyelid imaging
Artificial intelligence
INTRODUCTION
Since its introduction for the 1st time in the 1950s, artificial intelligence (AI) has captured the imagination of one and all.[1] With continuous technological developments and AI becoming a part of our everyday lives through various applications,[2,3] the fascination with AI has only increased further. AI has become a part of the healthcare sector, in the form of advanced machinery[4,5] and various software.[6,7] The applications of AI in the field of medicine are through its advanced forms, machine learning (ML), and deep learning (DL). While ML signifies a machine’s capacity to learn without receiving overt commands, DL is a further evolved category of ML which empowers AI systems to make choices by means of artificially created neural networks. Convolutional neural network (CNN) is a DL architecture that can process and learn directly from data. The ability to process huge databases, recognize patterns in images, and formulate predictive models puts AI in a great position to benefit medicine, especially technology and image-oriented branches like ophthalmology. A variety of imaging modalities are used by ophthalmologists, such as external photography, slit-lamp photography, fundus images, computed tomography (CT), and magnetic resonance imaging (MRI). With the continuous blending of technology and medicine, multiple researches have employed AI in ophthalmology.[8-11] Through this manuscript, we summarize the use of AI in the subspecialty of oculoplastic and reconstructive surgery.
PTOSIS
Ptosis is a common presentation in the oculoplastics clinic but a mild ptosis may be missed by a physician or general ophthalmologist. Malposition of the eyelid may be a presentation of an underlying disorder such as ptosis in myasthenia gravis/third nerve palsy or eyelid retraction in thyroid ophthalmopathy. Thus, detection and prompt referral to a specialist may be key to early diagnosis. The dynamic nature of eyelid and subjective variation among clinicians may be another factor that hinders an early diagnosis. AI can provide an innovative solution and become a useful tool for general practitioners. Van Brummen et al.[12] used AI to determine static and dynamic measurements of eyelid parameters such as mean reflex distance (MRD), canthal height, and intercanthal distance. Static measurements were performed from digital photographs while dynamic measurements were performed from a smartphone-captured video of a natural blink. ResNet50 and UNet-style softwares were used for segmentation of photographs. The measurements by AI had comparable accuracy to human segmentation. Thomas et al.[13] used OpenFace AI software to perform automated measurements of eyelid palpebral aperture in ptosis patients. The results were comparable to that of manual measurement by clinicians. Chen et al.[14] performed eyelid parameter measurements including MRD1, MRD2, and levator muscle action by employing medical AI assistant AI system using a smartphone. The calculations performed by CNN showed a comparable correlation to human performance. A tablet device was used by Tabuchi et al.[15] to capture photographs in their study. AI software MobileNetV2 CNN used in this research could distinguish ptosis patients from normal subjects with high accuracy. Hung et al.[16] showed that a trained AI model VGG-16 CNN outperformed non-ophthalmologist physicians in detecting ptosis from digital photographs. AI could deliver a higher sensitivity and specificity with better accuracy than human examiners. Song et al.[17] developed an AI decision-making software to choose the type of ptosis surgery. A gradient-boosted decision tree recognized seven facial features to capture 2D and 3D data from photographs. The AI system processed data to determine whether a subject had ptosis and then differentiated ptosis into two categories, based on recommended surgical approach (frontalis suspension or levator muscle surgery). When 2D and 3D measurements were combined, a higher accuracy could be achieved.
SURGICAL RECONSTRUCTION
Orbital reconstruction is conventionally performed either with autografts (bone or cartilage grafts) or alloplastic implants. However, designing an autograft according to the desired shape/size is difficult while alloplastic implants such as titanium are mostly flat. These limitations can be overcome by designing customized implants for patients using 3D reconstruction of the images [Figure 1] followed by 3D printing technology. By “mirroring” the normal side from an orbital CT scan, a personalized implant can be created. These “patient-specific implants (PSIs)” follow a “lock and key” mechanism and can be used for orbital reconstruction in a variety of orbital disorders [Figure 2]. Habib and Yoon[18] reported the use of PSIs for revision orbital reconstruction in eight patients with good functional and aesthetic outcomes. Surgical reconstruction of the eyelid after tumor excision may pose a challenge to the surgeon. Decision regarding the timing of surgery and knowledge about the expected complexity can prepare a surgeon for better patient management. Tan et al.[19] developed an AI-based decision tree model to predict the expected surgical reconstruction complexity after the excision of eyelid tumor. A ML-based software applied AI algorithms to clinical photographs to predict the surgical complexity of a case. The study reported that Naive Bayesian model could best predict the surgical complexity while the ADTree model was capable of classifying cases that were at high risk of complex reconstructions. Computer-assisted navigation guidance is another useful adjunct in surgical reconstruction and has been discussed later.

- Cat 3D reconstruction of orbital fracture.

- “Lock and key” type fitting by pre-operative mirroring from normal side.
DETECTION OF ORBITAL FRACTURES
Inception V3 is a CNN that was utilized by Li et al.[20] for detection of orbital blow-out fractures. Radiological details from CT scans were extracted by Inception V3, followed by processing probability values by XGBoost ML model. Following segmentation and annotation processes, the AI system classified images into “fracture” and “non-fracture” categories. This DL software showed an accuracy of 92% in detection of blow-out fractures.
COSMETIC EYELID SURGERY
For comparison of therapeutic effects of autogenous fat filling (AFF) with conventional double eyelid surgery, a denoising AI algorithm was applied to CT scan images by Fang et al.[21] Symmetric extended convolutional residual network used in the study was found to have better performance than traditional CNN systems. It could maintain the details obtained from CT images, while removing artifacts. A lower complication rate and higher efficacy were noted in AFF using this AI algorithm.
ASSESSING CHANGES AFTER EYELID SURGERY
The changes in eyelids with ptosis and deep superior sulcus after levator muscle resection surgery were documented by Mawatari et al.[22] using ImageJ software. This AI software performed automated calculations of area under the eyelid sulcus (AES) from black and white clinical images of patients. A quantitative analysis of MRD and AES showed significant improvement after surgery at 3 monthly visits.
AI IN INTRAOCULAR AND PERIOCULAR CANCER
An AI-based eyelid tumor detection system (ETDS) was developed by Li et al.[23] for detection of eyelid malignancies. Faster region-based CNNs were utilized in this study. The ETDS system performed identification and cropping out of tumor area from clinical photographs of eyelids. The eyelid lesions were highlighted by the use of heatmaps and four AI algorithms were trained on the images. DenseNet121 algorithm performed the best at distinguishing malignant from benign lesions. Interestingly, this study concluded that AI was more sensitive in diagnosing eyelid malignancies than junior as well as senior ophthalmologists and was comparable to oculoplastics experts. AI has also been used by researchers to identify retinoblastoma (RB) from fundus images. Extreme learning mechanism (ELM) is a fast-learning artificial neural network that was used by Jaya et al.[24] for detection of RB from fundus photographs. Green channel grayscaling and contrast sketching were performed to enhance image quality, followed by segmentation and feature extraction from fundus images. The ELM software was then applied to identify RB and normal fundus images. In this study, AI showed an accuracy of 92% at identification of RB cases. Kaliki et al.[25] developed an AI algorithm for detecting and grouping intraocular RB. Open CV technique and DL algorithms were used to extract features and identify RB as well as normal fundus photographs from Retcam imaging system. A two-step method was used to detect tumor seeds. The AI system was trained to identify the number of tumors, optic disk, tumor seeds, and size of tumor. A sensitivity ranging from 63% to 100% and specificity ranging from 91% to 100% was noted across the various groups of RB.
THYROID ORBITOPATHY
Orbital CT scan is a common imaging modality for studying orbital soft-tissue changes in thyroid associated orbitopathy (TAO). Song et al.[26] applied a 3D ResNet AI model on CT scan images for screening TAO patients. They used two datasets for training the AI model on 3D orbital CT scans and two datasets for evaluation of the model. AI achieved a sensitivity of 87.33% and specificity of 84% in detecting TAO from CT scan images, thus, proving to be a promising screening tool.
NAVIGATION GUIDANCE IN SURGERIES
The orbit, apart from the eyeball contains several vital structures in a compact bony space and thus, orbital surgeries can be extremely challenging. Intraoperative navigation is much like global positioning system tracking and helps in identification of landmarks, especially when the anatomy is distorted. CT, MRI, and magnetic resonance angiography scans can be loaded into the navigation system preoperatively to identify landmarks and target areas in complex orbital surgeries [Figure 3]. The intraoperative anatomy can be correlated to pre-surgical scans and the target areas can be approached accurately with the help of navigation stylet, which provides real-time tracking to the surgeon. A variety of orbital conditions can be managed effectively with navigation-guided surgery (NGS).[27] In post-traumatic orbital reconstruction, virtual planning can be performed by “mirroring” the normal side onto the affected side for accurate reconstruction. Navigation guidance can help delineate orbital tumors and margins of resection, without unnecessary manipulation. In traumatic optic neuropathy cases, NGS is a useful aide during optic canal decompression for localizing the optic canal and removing the bony fragments impinging the optic nerve [Figure 4]. The use of NGS for recognizing exact bone landmarks during bone decompression in TAO cases has also been described.[28]

- Application of real time navigation in orbit.

- Application of global positioning system for precise intraoperative localization of lesion.
CONCLUSION
The future lies in understanding the intersection of technology with oculoplastics practice for improving patient care. Developing and refining AI is necessary to put oculoplastics surgeons at the vanguard of technological revolution. AI has a huge potential to become an important tool in the armamentarium of not only general practitioners but also specialists.
Declaration of patient consent
Patient’s consent not required as there are no patients in this study.
Conflicts of interest
There are no conflicts of interest.
Financial support and sponsorship
Nil.
References
- Integrating artificial and human intelligence: A partnership for responsible innovation in biomedical engineering and medicine. OMICS. 2020;24:247-63.
- [CrossRef] [PubMed] [Google Scholar]
- Music Personalization at Spotify In: Paper Presented at: Proceedings of the 10th ACM Conference on Recommender Systems. 2016.
- [CrossRef] [Google Scholar]
- Available from: https://master-artificialintelligence.com/aialexa-and-siri [Last accessed on 2023 Apr 06]
- Robot companions and ethics a pragmatic approach of ethical design. J Int Bioethique. 2013;24:49-58.
- [CrossRef] [PubMed] [Google Scholar]
- Artificial intelligence in medicine: Current trends and future possibilities. Br J Gen Pract. 2018;68:143-4.
- [CrossRef] [PubMed] [Google Scholar]
- Artificial intelligence in radiology: Friend or foe? Where are we now and where are we heading? Acta Radiol Open. 2019;8:205846011983022.
- [CrossRef] [PubMed] [Google Scholar]
- Overview of artificial intelligence in medicine. J Family Med Prim Care. 2019;8:2328-31.
- [CrossRef] [PubMed] [Google Scholar]
- Improved automated detection of diabetic retinopathy on a publicly available dataset through integration of deep learning. Invest Ophthalmol Vis Sci. 2016;57:5200-6.
- [CrossRef] [PubMed] [Google Scholar]
- A deep learning algorithm for prediction of age-related eye disease study severity scale for age-related macular degeneration from color fundus photography. Ophthalmology. 2018;125:1410-20.
- [CrossRef] [PubMed] [Google Scholar]
- Personalized prediction of glaucoma progression under different target intraocular pressure levels using filtered forecasting methods. Ophthalmology. 2018;125:569-77.
- [CrossRef] [PubMed] [Google Scholar]
- Artificial intelligence in cataract: What's new? Glob J Cataract Surg Res Ophthalmol. 2022;1:36-42.
- [CrossRef] [Google Scholar]
- PeriorbitAI: Artificial intelligence automation of eyelid and periorbital measurements. Am J Ophthalmol. 2021;230:285-96.
- [CrossRef] [PubMed] [Google Scholar]
- An artificial intelligence approach to the assessment of abnormal lid position. Plast Reconstr Surg Glob Open. 2020;8:e3089.
- [CrossRef] [PubMed] [Google Scholar]
- Smartphone-based artificial intelligence-assisted prediction for eyelid measurements: Algorithm development and observational validation study. JMIR Mhealth Uhealth. 2021;9:e32444.
- [CrossRef] [PubMed] [Google Scholar]
- Developing an iOS application that uses machine learning for the automated diagnosis of blepharoptosis. Graefes Arch Clin Exp Ophthalmol. 2022;260:1329-35.
- [CrossRef] [PubMed] [Google Scholar]
- An outperforming artificial intelligence model to identify referable blepharoptosis for general practitioners. J Pers Med. 2022;12:283.
- [CrossRef] [PubMed] [Google Scholar]
- A clinical decision model based on machine learning for ptosis. BMC Ophthalmol. 2021;21:169.
- [CrossRef] [PubMed] [Google Scholar]
- Patient specific implants in orbital reconstruction: A pilot study. Am J Ophthalmol Case Rep. 2021;24:101222.
- [CrossRef] [PubMed] [Google Scholar]
- A practical decision-tree model to predict complexity of reconstructive surgery after periocular basal cell carcinoma excision. J Eur Acad Dermatol Venereol. 2017;31:717-23.
- [CrossRef] [PubMed] [Google Scholar]
- Deep convolutional neural networks for automatic detection of orbital blowout fractures. J Craniofac Surg. 2020;31:400-3.
- [CrossRef] [PubMed] [Google Scholar]
- Removal of autogenous fat filling in double eyelid operation by artificial intelligence (AI) algorithm-based computerized tomography (CT) image features. Sci Program. 2022;2022:9367906.
- [CrossRef] [Google Scholar]
- Changes in sunken eyes combined with blepharoptosis after levator resection. Plast Reconstr Surg Glob Open. 2017;5:e1616.
- [CrossRef] [PubMed] [Google Scholar]
- Artificial intelligence to detect malignant eyelid tumors from photographic images. NPJ Digit Med. 2022;5:23.
- [CrossRef] [PubMed] [Google Scholar]
- Identification of retinoblastoma using the extreme learning machine. J Phys Conf Ser. 2019;1235:12057.
- [CrossRef] [Google Scholar]
- Artificial intelligence and machine learning in ocular oncology: Retinoblastoma. Indian J Ophthalmol. 2023;71:424-30.
- [CrossRef] [PubMed] [Google Scholar]
- Artificial intelligence CT screening model for thyroid-associated ophthalmopathy and tests under clinical conditions. Int J Comput Assist Radiol Surg. 2021;16:323-30.
- [CrossRef] [PubMed] [Google Scholar]
- Computer-assisted navigation in orbitofacial surgery. Indian J Ophthalmol. 2019;67:995-1003.
- [CrossRef] [PubMed] [Google Scholar]
- A quantitative approach to orbital decompression in Graves' disease using computer-assisted surgery: A compilation of different techniques and introduction of the “temporal cage”. J Oral Maxillofac Surg. 2012;70:1152-60.
- [CrossRef] [PubMed] [Google Scholar]